In the digital age, machine learning (ML) stands as a revolutionary force, driving innovations that were once the stuff of science fiction. From enhancing medical diagnostics to powering self-driving cars, machine learning is reshaping industries and creating a high demand for skilled professionals. This blog explores the vast career opportunities in machine learning, guiding you through the educational paths, required skills, job roles, and the dynamic landscape of industries transformed by this powerful form of artificial intelligence (AI).
Importance of machine learning in the modern tech landscape
Machine learning (ML) has become a cornerstone of the modern technology landscape, fundamentally altering how industries operate and innovate. By enabling computers to learn from and interpret data without explicit programming, ML allows for more efficient data processing, predictive accuracy, and decision-making capabilities across diverse sectors.
In today’s data-driven world, the ability to quickly analyze large datasets and derive meaningful insights is invaluable. Machine learning excels in this regard, offering solutions that can adapt and improve over time. This adaptability is crucial in fields such as healthcare, where ML models help predict patient outcomes and tailor treatments, and in finance, where they detect fraudulent transactions in real-time.
Furthermore, ML is pivotal in developing emerging technologies like autonomous vehicles and intelligent robotics, pushing the boundaries of what machines can achieve. Its role in enhancing user experiences through personalized recommendations on platforms like Netflix and Amazon underscores its impact on everyday life.
As AI continues to evolve, the importance of machine learning will only grow, representing a key driver of innovation and competitive advantage. This makes understanding and leveraging ML essential for businesses looking to succeed in an increasingly automated and intelligent future.
Brief overview of the rise of AI and machine learning
The rise of artificial intelligence (AI) and machine learning (ML) marks one of the most significant technological revolutions of our time. Initially rooted in academic theory and experimentation in the mid-20th century, the concepts of AI and ML began to take shape with the development of algorithms that could simulate human decision-making processes and improve through experience.
A pivotal moment in this journey was the introduction of neural networks in the 1980s, which mimicked the human brain’s ability to recognize patterns and learn from data. However, it wasn’t until the last decade, with the explosion of big data and advancements in computing power, that AI and ML began to profoundly impact industries and daily life.
Today, machine learning algorithms drive a wide range of applications, from the predictive text features in smartphones to complex decision-making systems in autonomous vehicles. Companies across sectors leverage ML to optimize operations, enhance customer interactions, and unlock new business opportunities through predictive analytics.
The ongoing advancements in deep learning and neural networks continue to push the boundaries, suggesting that the influence of AI and ML is just beginning. This trajectory not only reshapes how businesses operate but also how society adapts to these evolving technologies.
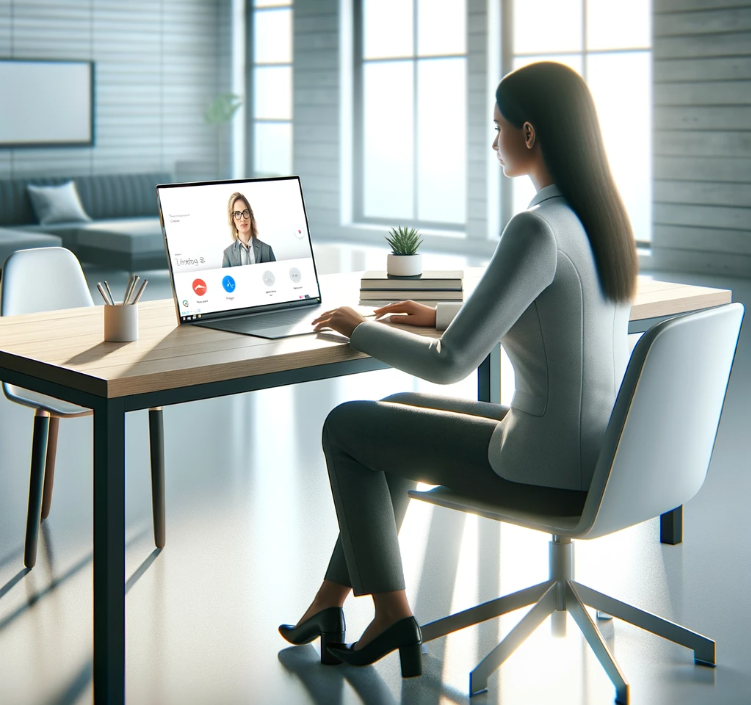
Educational Pathways
Embarking on a career in machine learning requires a solid educational foundation in key areas such as statistics, computer science, and data analysis. Pursuing specific degrees and certifications can equip aspiring professionals with the necessary skills and knowledge to excel in this dynamic field.
Degrees
- Bachelor’s Degree in Computer Science: Provides a comprehensive foundation in programming, algorithms, and systems analysis. It’s an essential stepping stone for understanding the technical aspects of machine learning.
- Master’s Degree in Data Science or Machine Learning: Offers specialized knowledge in machine learning algorithms, deep learning, and predictive analytics. This degree typically involves practical projects and research in machine learning.
- Ph.D. in Artificial Intelligence: Ideal for those interested in research or academic careers. This advanced degree focuses on innovative machine learning techniques and their applications.
Certifications
- Google Professional Machine Learning Engineer: Validates skills in designing, building, and running machine learning models using Google Cloud technologies.
- IBM Data Science Professional Certificate: Covers data science and machine learning fundamentals using Python and includes hands-on projects to build models.
- Certified Analytics Professional (CAP): Focuses on the end-to-end analytics process and how machine learning fits within broader strategic objectives.
These educational paths provide both theoretical understanding and practical experience, making them invaluable for anyone looking to make a mark in the field of machine learning.
Core Skills Required
In the rapidly evolving field of machine learning, possessing a strong set of technical skills is essential for success. Key among these are proficiency in programming languages, a deep understanding of statistics, and robust data analysis capabilities.
Programming Languages: Python and R are the cornerstone languages for machine learning. Python boasts an extensive ecosystem of libraries such as NumPy, pandas, and TensorFlow, making it ideal for both beginners and seasoned professionals. R, on the other hand, is highly regarded for statistical analysis and graphical representations, favored especially in academia and research.
Statistics: A solid grasp of statistics is crucial for understanding data behaviors, which underpin all machine learning algorithms. Knowledge areas such as probability distributions, statistical significance, and hypothesis testing are foundational in developing models that are both accurate and reliable.
Data Analysis: The ability to manipulate and extract insights from data is a critical skill in machine learning. It involves cleaning, summarizing, and interpreting data, ensuring that the input data used in machine learning models is of high quality and relevant.
Together, these skills form the backbone of effective machine learning practice. They enable practitioners to build sophisticated models that can predict trends, automate tasks, and drive decision making, transforming raw data into valuable insights.
While technical prowess is vital in machine learning, the importance of soft skills cannot be underestimated, especially when it comes to problem-solving, critical thinking, and effective communication. These skills are crucial in translating technical expertise into real-world impacts.
Problem-solving is at the heart of machine learning. Professionals in the field are often faced with complex challenges, such as optimizing algorithms, improving model accuracy, or dealing with vast datasets. Effective problem-solving involves a creative approach to breaking down complex issues into manageable parts and devising efficient solutions.
Critical thinking is essential for evaluating the validity of models and interpreting their outputs. Machine learning practitioners must critically assess whether their models are biased, whether the data adequately represents the problem, and how the outcomes affect real-world decisions. This requires a keen, analytical mindset that questions assumptions and tests theories systematically.
Effective communication is crucial, especially when explaining technical details to non-experts or stakeholders. Machine learning professionals must be able to articulate complex concepts clearly and convey the significance and limitations of their findings. This ensures that their work is understood and appreciated, facilitating better decision-making and fostering collaborative efforts.
Together, these soft skills amplify the technical capabilities of machine learning professionals, enabling them to lead projects effectively and drive innovation in their organizations.
Emerging Job Roles in Machine Learning
- Data Scientist: Data Scientists are invaluable in industries like finance, healthcare, consulting, technology, and anywhere data needs to be analyzed to enhance performance and outcomes. They must possess a strong foundation in computer science, statistics, and mathematics, along with excellent communication skills to interpret and convey data-driven insights.
- Machine Learning Engineer: A Machine Learning Engineer is a specialized role that focuses on researching, building, and designing self-running artificial intelligence (AI) systems to automate predictive models. Machine Learning Engineers create algorithms that allow computers to perform tasks without explicit instructions, relying on patterns and inference instead.
- AI Research Scientist: An AI Research Scientist investigates new methods and algorithms to improve and advance the field of artificial intelligence. Their work is often foundational, focusing on understanding and designing the computational mechanisms underlying intelligent behavior.
- Robotics Engineer: A Robotics Engineer designs, builds, and tests robots that are efficient and safe to operate while capable of performing tasks that are typically done by humans. Their work involves a combination of several disciplines including computer science, electrical engineering, and mechanical engineering.
Industries Leveraging Machine Learning
- Healthcare: improving diagnostics and patient care.
- Finance: risk assessment, fraud detection.
- Automotive: self-driving car technology.
- Retail: customer behavior analysis and personalized marketing.
Challenges in a Machine Learning Career
- Keeping up with rapidly evolving technologies.
- Ethical considerations in AI development.
Company and Package offers
Machine learning is a rapidly growing field that offers lucrative salary packages across various industries and locations. Some top tech companies are known for their competitive salaries, significantly influencing potential earnings in this career.
In the United States, for instance, machine learning engineers can expect a broad range of salaries depending on their experience and the specific industry they work in. Entry-level positions typically offer salaries ranging from around $96,000 to $132,000 annually, depending on the company and location. As machine learning engineers gain experience, their earning potential increases, with mid-career professionals earning between $99,000 and $180,000, and those in late-career stages potentially making between $115,000 and $204,000 annually.
Top-paying companies like Cruise, Stripe, Wish, Meta, and Google offer substantial packages for machine learning roles. For example, Cruise offers around $273,319, Stripe around $272,258, and Google around $258,102.
Globally, salaries also vary significantly. Machine learning engineers in Switzerland, the United States, and Australia are among the highest earners. However, in tech hubs like San Francisco, salaries can reach up to $172,678, while in smaller cities like Austin, Texas, the average might be around $133,251.